Researchers found that in some stores, highly processed foods were the only option in some categories
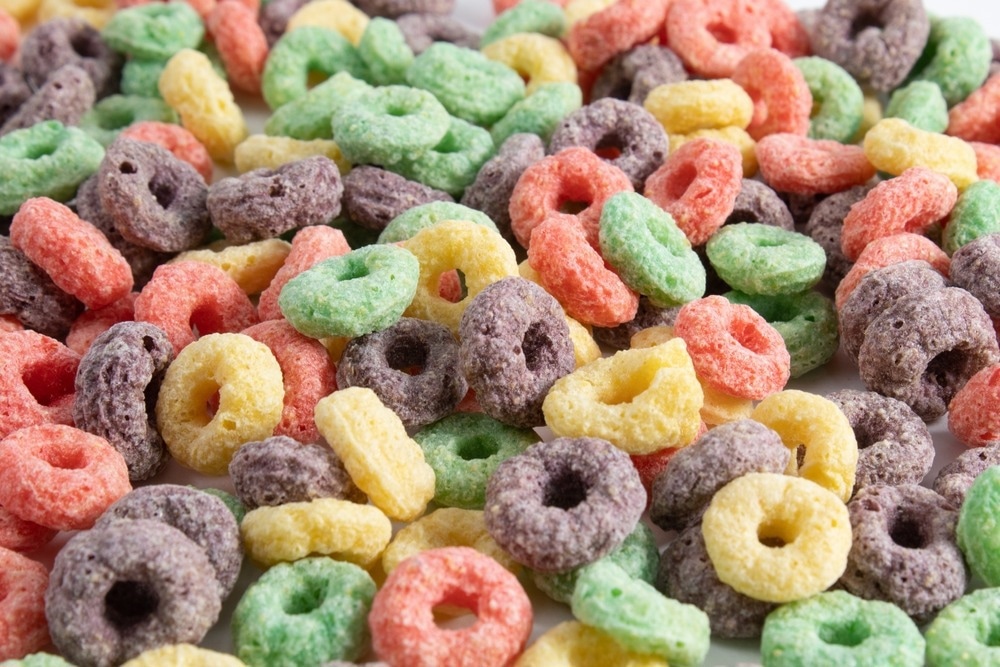
A recent Nature Food study used machine learning techniques to analyze over 50,000 products from major US grocery store websites, developing the GroceryDB database, which facilitates consumer decision-making and informs public health initiatives.
Quantifying the extent of food processing in grocery stores
Research has shown the adverse health implications of reliance on ultra-processed food (UPF), which contributes up to 60% of total calorie intake in developed countries. Much of UPF reaches consumers through grocery stores, which motivates questions surrounding quantifying the extent of food processing in the food supply, methods to be used, and alternatives to reduce UPF consumption.
Measuring the degree of food processing is not straightforward because food labels often contain mixed and unclear messages, leaving room for ambiguity and differences in interpretation. Therefore, scientists have been advocating for a more objective definition of the degree of food processing based on biological mechanisms.
Furthermore, owing to the large-scale and complex data in question, artificial intelligence (AI) methodologies are increasingly being used to advance nutrition security.
About the study
Publicly accessible data on food products were compiled from the websites of the US’s leading grocery stores, Walmart, Target, and Whole Foods. The websites were navigated to identify specific food items, and consistency was ensured by aligning the classification systems used by each store.
The food labels were used to standardize nutrient concentrations, while FoodProX was used to assess each item’s degree of food processing. FoodProX is a random forest classifier that translates the combinatorial changes in the quantities of nutrients affected by food processing into a food processing score (FPro).
Extensive tests and validations on the stability of FPro were performed. The final score was contingent on the probability of observing the overall pattern of nutrient concentrations in unprocessed food as opposed to UPF. The price per calorie variation at various levels of food processing was computed using robust linear models with Huber’s t-norm.
Study findings
Leveraging the machine learning classifier FoodProX, the GroceryDB database assigned an FPro score to all food items. Across all three supermarkets, the FPro distribution was similar, and the results suggested that low FPro foods (minimal processing) account for a relatively small fraction of grocery store inventory. Most items were in the high FPro or UPF category. The low FPro items account for a proportionally greater fraction of actual purchases, showing a mismatch between sales data and available food options.
Some differences across stores were noted, i.e., Whole Foods offers fewer ultra-processed options, while Target offers a high proportion of high FPro food items. Low FPro variation was noted in categories like jerky, popcorn biscuits, mac and cheese, chips, and bread, highlighting limited consumer choice in these segments. This was not the case in other categories, such as cereals, pasta noodles, milk and milk substitutes, and snack bars, where consumers had more choices. Moreover, the distribution of FPro in GroceryDB and the latest USDA Food and Nutrient Database for Dietary Studies (FNDDS) was similar.
Concerning the relation between price and calories, a 10% increase in FPro resulted in an 8.7% decrease in the price per calorie of products across all categories in GroceryDB. The food category was important in the relationship between FPro and price per calorie, with most processed foods likely being cheaper per calorie than the minimally processed alternatives. The relationship between milk and milk-substitute category and FPro showed an increasing trend.
Regarding store heterogeneity in the same food category, the analysis showed that cereals sold at Whole Foods typically contain fewer artificial and natural flavors, less sugar, and fewer added vitamins relative to Walmart and Target. The brands offered by each store could also explain the heterogeneity, with Whole Foods relying on suppliers different from Target and Walmart.
Some food categories, such as pizza, popcorn, and mac and cheese, are highly processed in all stores. As per GroceryDB, Whole Foods offers a wider FPro range of cookies and biscuits for consumers to choose from, whereas Target and Walmart have identical and narrower ranges of FPro scores.
An ingredient FPro (IgFPro), ranging from 0 (unprocessed) to 1 (ultra-processed), was calculated to rank ingredients based on their contribution to the degree of processing of the final product. By analyzing a variety of food items, it was shown that not all ingredients contribute equally to the amount of processing, and food products with more complex ingredient lists tend to be more processed.
Conclusions
In summary, this work uses machine learning techniques to model the chemical complexity of food items offered by some leading supermarkets in the US. GroceryDB and FPro offer a data-driven approach for consumers to identify similar but less processed alternatives across a range of categories.
Journal reference:
- Ravandi, B., Ispirova, G., Sebek, M., Mehler, P., Barabási, A., & Menichetti, G. (2025) Prevalence of processed foods in major US grocery stores. Nature Food, 1-13. https://doi.org/10.1038/s43016-024-01095-7